This article has been penned by Swapan Rajdev, Co-Founder & CTO of Haptik
Chatbots and virtual assistants have been in the news in a big way for the past few days. The reason? One word: Meena.
In a research paper titled Towards an Open Domain Chatbot, Google presented Meena, a “conversational agent that can chat about…anything”.
According to the Google researchers’ who worked on the project, Meena stands in contrast to the majority of current chatbots, that tend to be highly specialized within a particular domain, and perform well as long as users do not stray too much from expected usage. Open-domain chatbots, on the other hand, theoretically have the ability to converse with a user about anything they want.
In practice, however, most current open-domain chatbots often simply do not make sense. At worst, they say things that are inconsistent with what has been said previously, or which indicate a lack of common sense or basic knowledge about the world. At best, they can say something like “I don’t know” – a sensible response to any query, but one which does not address the specific needs of the user.
This is the gap that Google aims to address with Meena, which they claim has come closer to simulating the experience of a conversation with an actual human being than any other state-of-the-art chatbot to date.
Meena is undoubtedly a game-changer in the Conversational AI space. But what lessons does it hold for enterprises that have implemented, or plan to implement, conversational solutions? Can brands look forward to the day when they have their own “Meena’s” chatting away to customers and achieving hitherto unknown milestones in customer engagement? Read on as we unpack Meena and attempt to answer those questions.
The conversational experience users always wanted (but never got)
When it comes to chatbots, the expectation has always been that one can interact with them much like one does with a fellow human – asking them anything under the sun and receiving an engaging response that makes perfect sense. But reality has thus far fallen short of those expectations.
Meena certainly brings us a lot closer to bridging that gap between expectation and reality. It has been described as a multi-turn and open-domain chatbot – both terms being key to understanding its capabilities. Open-domain essentially means that there are no restrictions on the topics that can be discussed with the chatbot. Multi-turn means that the chatbot is capable of engaging in a conversation that involves back-and-forth between participants. Both of these form the backbone of Meena’s ability to effectively simulate human conversation.
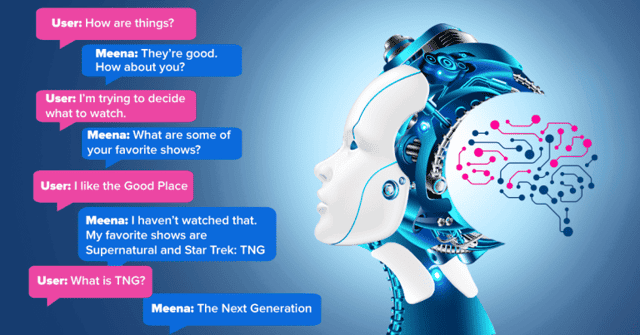
As an open-domain and multi-turn chatbot, Meena is capable of effectively simulating human conversation. Source: Google Blog
To mimic human conversation, it isn’t enough for a chatbot to say something that makes sense. It’s equally important to make sense in context. As we discussed at the start, a chatbot saying “I don’t know” in response to a user’s query technically makes sense, but it does not address the specific query the user had. This response could indicate one of two possibilities – a) that the chatbot did not understand the user’s query, or b) that the chatbot understood the query but genuinely did not know the answer. It is important to differentiate between the two, to gauge how good the chatbot really is at understanding.
This becomes very important when it comes to the chatbot’s ability to effectively simulate human conversation. If the chatbot responds “I don’t know” to certain queries that it simply does not know the answer to, it is akin to a person genuinely not knowing the answer to certain questions. But if a chatbot repeatedly replies “I don’t know” to even basic queries that it would reasonably be expected to know the answers to, then that would shatter the illusion of human-like conversation – ultimately having an adverse impact on end-user experience.
Google has, in fact, introduced a new metric to demonstrate how good Meena is at simulating human conversation – the Sensibleness and Specificity Average (SSA). To determine the SSA of Meena, evaluators tested Meena, as well as a few other open-domain chatbots (Mitsuku, Xiaoice, Cleverbot, DialoGPT), assessing every response on the basis of two questions – “does it make sense?” and “is it specific?”.
Meena’s achieved an SSA score of 79%, leaps and bounds ahead of the other chatbots tested (its closest competitor, five-time Loebner Prize winner Mitsuku, scored 56%). To put this into perspective, the SSA score of an average person was found to be 86% – a mere 7% above Meena’s score. This certainly places Meena’s ability to ‘speak like a human’ into stark relief!
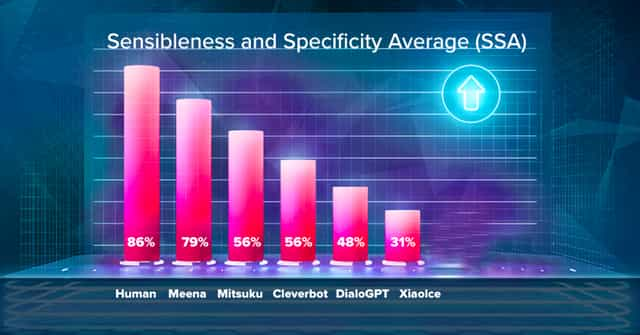
Meena’s high SSA (Sensibleness and Specificity Average) score reflects its superior ability to engage in human-like conversation. Source: Google Blog
Meena has undoubtedly come closer than any of its peers to fulfilling the long-standing expectation of being able to talk to a chatbot about ‘anything’. Let us now examine another crucial aspect of Meena that should be significant to anyone observing the Conversational AI space.
Conversational data is the key
An open-domain chatbot’s ability to engage in free-flowing conversation with a user depends to a large extent on the dataset used to train it. The larger and more varied the dataset the AI is trained on, the greater the scope of the queries it is able to address. And Meena has certainly outclassed its competition in terms of the sheer quantum of data used to train it.
Meena has 2.6 billion parameters and was trained on 341 GB of textual data (comprising 40 billion words), derived from public-domain social media conversations. Apart from the staggering amount of data involved, the key point to note here is that the data Meena was trained on was conversational data i.e. conversations and messages written by real human beings.
In an enterprise context, as we’ve discovered at Haptik over the years, conversational data sourced from interactions between real customers and support agents or sales assistants is particularly crucial. The best way to simulate an engaging and personalized customer support or sales experience is to train your Conversational AI on data from real customer interactions. Meena’s vastly superior capabilities certainly highlight the necessity of this approach to training chatbots.
What does Meena mean for enterprise virtual assistants?
All the buzz created by Meena over the past few weeks has no doubt generated a lot of interest across industries about the enterprise applications of this highly sophisticated and interactive chatbot model.
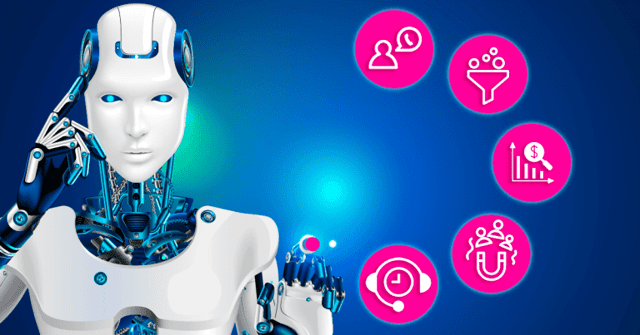
There’s certainly a lot about Meena that would be immensely beneficial to a brand looking for innovative ways to engage customers. The ability to engage in free-flowing conversation brings a naturalistic ‘human’ touch to interactions between customers and virtual assistants, significantly enhancing customer experience. Haptik’s study on Virtual Assistant Personality Preference last year demonstrated the concrete impact that the chatbot personality can have on customer experience (with 67% of respondents expressing a preference for assistants with a more friendly personality). A chatbot with Meena’s capabilities would certainly up the ante when it comes to exhibiting distinct virtual personalities.
“Humanizing computer interactions, improving foreign language practice, and making relatable interactive movie and videogame characters” are some of the possible applications of the Meena chatbot model, according to Google. It wouldn’t be a stretch to add “more engaging, interactive and human-like virtual sales clerks and customer support agents” to that list!
So, how soon can enterprises get their own “Meena’s”?
Well, the realistic answer is – it will take a little time.
While Meena is definitely a giant leap in the right direction for Conversational AI, implementing a virtual assistant solution of equal sophistication is a somewhat challenging prospect for most enterprises at present.
To begin with, a Meena-like enterprise assistant would require to be trained on vast amounts of domain-specific conversational data. Acquiring data is not not particularly difficult, but cleaning it up to make it usable by Machine Learning (ML) models requires a fair amount of time and effort. Haptik is fortunate in this regard, as we have over 6 years worth of conversational data and have already invested a substantial amount of effort in preparing it for our ML.
However, access to vast amounts of conversational data is a relatively simpler barrier to overcome as compared to the hardware barrier. The processing power required to train a chatbot of Meena’s sophistication is tremendous, to put it mildly. It took Google’s researchers 2048 TPU cores to train Meena! Google has not officially released any figures, but there are estimates online which suggest that the cost would have easily run into over a million dollars. That being said, with ML models becoming more efficient and the hardware required becoming more cost-effective, this barrier is becoming less insurmountable
Looking ahead
Meena has definitely shown us all the road ahead, broadly speaking, for Conversational AI. From the emphasis on large data-sets for training (sourced from real human conversations), to simulating more free-flowing, interactive and human-like conversations, there’s a lot that chatbot developers, as well as enterprises implementing conversational solutions, can learn from Meena.
We at Haptik are excited by the possibilities that Meena has opened up in this space. Developments like these only encourage us to redouble our own efforts towards designing superior conversational experiences, and we look forward to showcasing some of our research soon.
